

Rapid and accurate disease type and disease area localization are crucial for disease prevention and control, achieving a high accuracy in the assessment of the severity of disease and the purpose of intelligent prevention. The primary applications of UAV in agriculture include mapping, spraying, pest detection, and other tasks. When employing UAVs, the wheat images are captured using an onboard camera. proposed a method combining unmanned aerial vehicle (UAV) technology and multispectral technology to detect the severity of wheat FHB with 98% accuracy. For instance, many researchers have gained considerable attention for the safety and convenience of UAV-based wheat disease detections. Many studies have also been conducted to find a quick and accurate way for FHB detection. Furthermore, the conventional approaches are ineffective in detecting large-scale disease. Chromatography and ELISA are highly accurate, sensitive, and dependable, but they typically require multiple processing steps, expensive equipment, and a long turnaround time. Visual inspection is convenient, but highly subjective, requiring various human, material, and financial resources based on morphological characteristics and experience. Visual inspection, chromatography, and enzyme-linked immunoassay (ELISA) are the conventional methods for detecting FHB. FHB is also one of the most devastating cereal diseases causing significant yield and quality reduction.

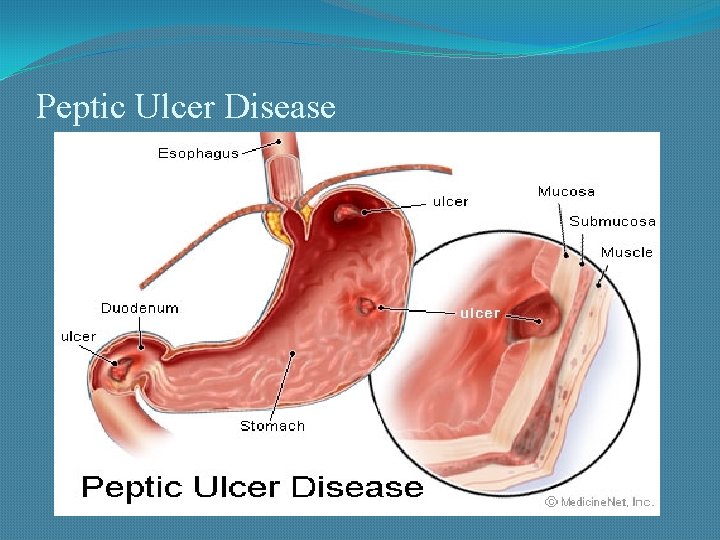
Processed products made from wheat kernels carrying these toxins likely lead to food poisoning and gradual decline of immunity and physical health. Meanwhile, several Fusarium alenol toxins are secreted. Diseased spikes reveal premature bleaching and generate shriveled kernels when FHB infects wheat kernels. Under normal circumstances, there is little or no FHB infection below 15 ☌, and the possibility of wheat infected with FHB increases as the temperature rises from 20 ☌ to 30 ☌. FHB mainly occurs in warm and humid environments. In many parts of the world, Fusarium head blight (FHB), often known as head scab, is one of the most severe and widespread wheat diseases caused by Fusarium graminearum and Fusarium asiaticum. Wheat proteins contain sufficient essential amino acids to meet daily requirements, while wheat grains provide a variety of vitamins, minerals, and other phytochemicals, significantly impacting human health. Wheat is one of the world’s most important staple foods, providing both calories and nutrients, and wheat-based foods are essential for food security of global population. Overall, the proposed model could be deployed on UAVs so that wheat ear FHB detection results could be sent back to the end-users to intelligently decide in time, promoting the intelligent control of agricultural disease. Meanwhile, the suggested model was scaled down to a fifth of the size of the state-of-the-art object detection models. The experimental results demonstrated that the proposed model performed admirably well in detecting FHB of the wheat ear, with an accuracy of 93.69%, and it was somewhat better than the MobileNetv2-YOLOv4 model (F1 by 4%, AP by 3.5%, Recall by 4.1%, and Precision by 1.6%). Finally, mixed-up and transfer learning schemes enhanced the model’s generalization ability. Furthermore, the loss function incorporated the focal loss to reduce the error caused by the unbalanced positive and negative sample distribution. Additionally, we employed the Complete Intersection over Union (CIoU) and Non-Maximum Suppression (NMS) to regress the loss function to guarantee the detection accuracy of FHB. Specifically, the backbone network Cross Stage Partial Darknet53 (CSPDarknet53) of YOLOv4 was replaced by a lightweight network, significantly decreasing the network parameters and the computing complexity. Our model utilized the You Only Look Once version 4 (YOLOv4) and MobileNet deep learning architectures and was applicable in edge devices, balancing accuracy, and FHB detection in real-time. Hence, this paper proposed a lightweight model for wheat ear FHB detection based on UAV-enabled edge computing, aiming to achieve the purpose of intelligent prevention and control of agricultural disease.
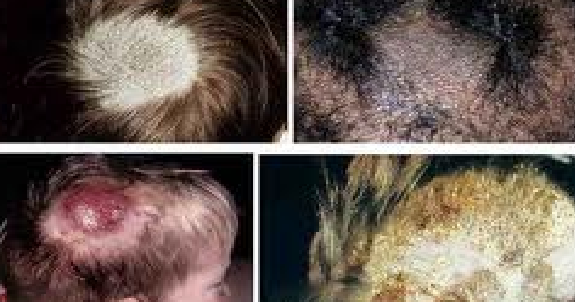
FHB detection tasks in agricultural production are currently handled by cloud servers and utilize unmanned aerial vehicles (UAVs). Detection of the Fusarium head blight (FHB) is crucial for wheat yield protection, with precise and rapid FHB detection increasing wheat yield and protecting the agricultural ecological environment.
